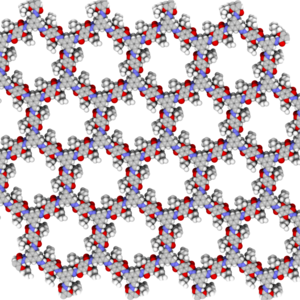
College of Engineering Unit:
Nanoporous materials (NPMs) leverage physical interactions in nanometer-scale pores to selectively adsorb and concentrate gasses, and find use in applications such as sensing and catalysis. Covalent organic frameworks (COFs) are a diverse subclass of modular NPMs, exceeding 560,000 discrete structures hypothesized in silico to date. However, the adsorption properties of these thousands of hypothetical structures are unknown. High-throughput molecular simulations can accelerate the discovery of performant NPMs, but are computationally expensive and often require days to complete. Employing machine learning can greatly reduce the computational cost of screening large databases of NPMs. Current popular machine learning techniques utilize partially observed target values or hand-crafted features, both of which are non-trivial to obtain for unobserved hypothetical structures. The cold-start problem, in which only the molecular structure of a material is known, is the primary inhibitor of existing methods. In this work, we design and train a message passing neural network (MPNN) to predict uptakes of CH4, H2O, H2S, Xe, Kr, CO2, N2, O2, and H2 at various temperatures and pressures. Using a set of 556 COFs, we train on a partially complete set of adsorption tasks and the bonding structure of the molecule as a graph. The learned representation from the bonding graph is used to impute the missing properties. Our MPNN can assign gas uptakes in COFs with high fidelity, with a Spearman’s rank correlation coefficient of ~0.78 across all targets. Furthermore, our model only requires the bonding graph as input during inference, thus overcoming the critical cold-start problem when a new unobserved material is introduced. While the performance of our model is competitive, further analysis is being conducted to improve the accuracy over other existing methods that leverage a more vast feature space as inputs. The concepts in our work can be applied broadly to the screening of many different unobserved materials and properties.
Project Communication Piece(s):
Attachment | Size |
---|---|
![]() | 2.24 MB |
![]() | 1.66 MB |